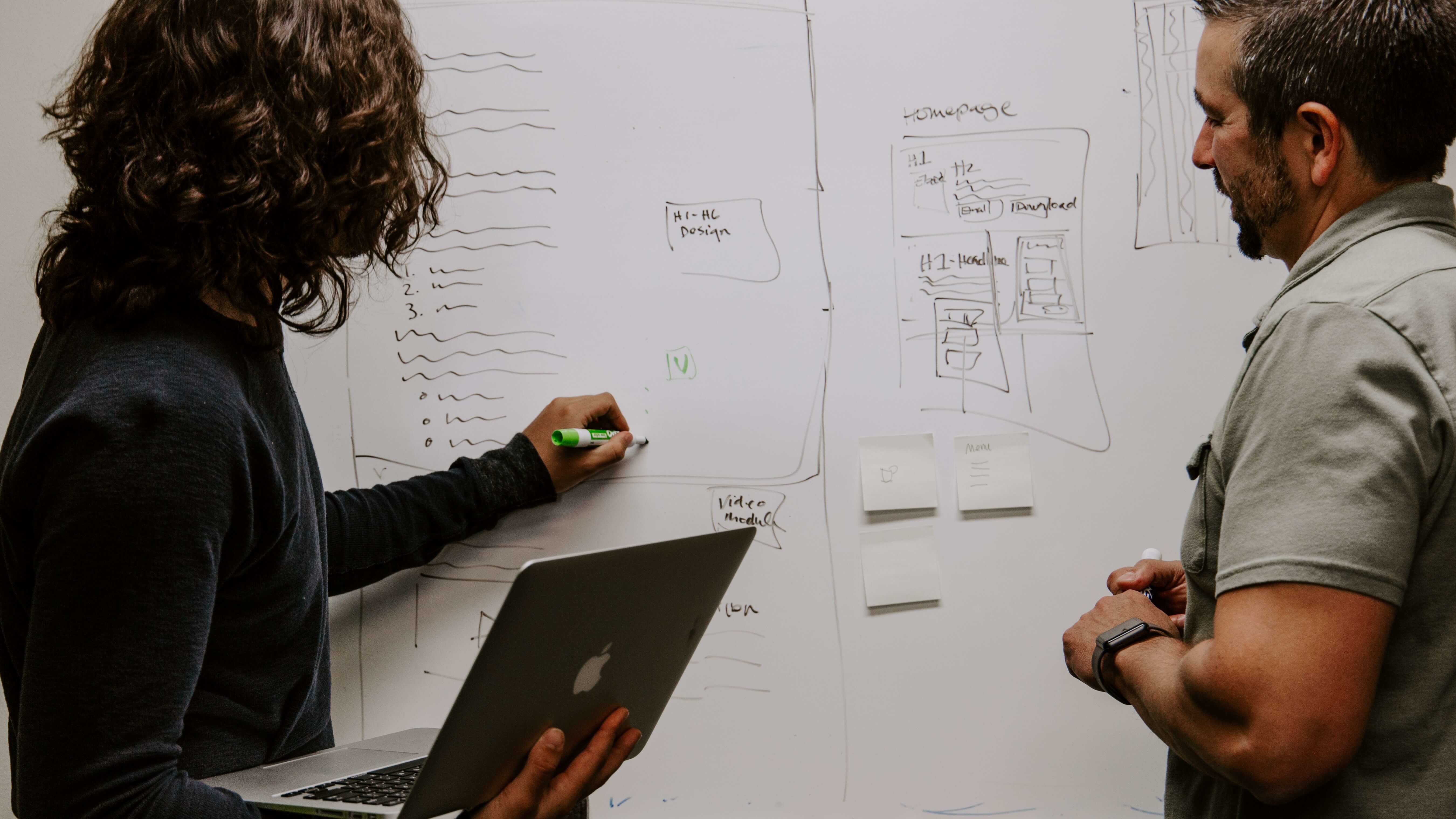
Enhancing strategic decisions with Data analytics
Challenges
Segment and competitor volume forecasts play an important role in reducing mismatched supply within the organization. In particular, procurement and production planning are affected.
However, there were three significant fundamental limitations for these forecasts:
- Low level of automation: The forecasts were manually created in Excel
- Number of data sources: Heavy focus was on a single external input source with limited forecast granularity
- Lack of suitable statistical prognosis model: Only standard linear statistical extrapolation was used.
Furthermore, forecasting granularity and scope was to be increased in the future. But that would have required too much effort in the existing environment.
That’s why rpc developed and implemented a data analytics solution to manage these problems.
Approach in detail
Definition of business objectives and analytics pilot goals
For the first step of developing a data analytics solution, business understanding was needed to determine business objectives, to assess the situation, to define analytics pilot goals, and to set up a project.
Data preparation and statistical modeling
Historical registration and sales data was pre-processed and additional data sources were included. Several kinds of autoregressive (ARIMA, ARIMAX, VAR), exponential smoothing (Holt Winters) and machine learning methods (Random Forest, Regression Tree) were implemented and evaluated to achieve the desired results.
Use of open source libraries
Well-established open source libraries were used as efficient, flexible tools for data analysis that can be integrated into existing IT-systems.
Created impact
Automated, data-driven volume forecasts lay the foundation for for better planning within the company.
68%
of forecasts have increased their accuracy
16
month timeframe for improved granularity
3
new markets in forecasting scope